Artificial Intelligence for Medical Data Science and Epidemiology
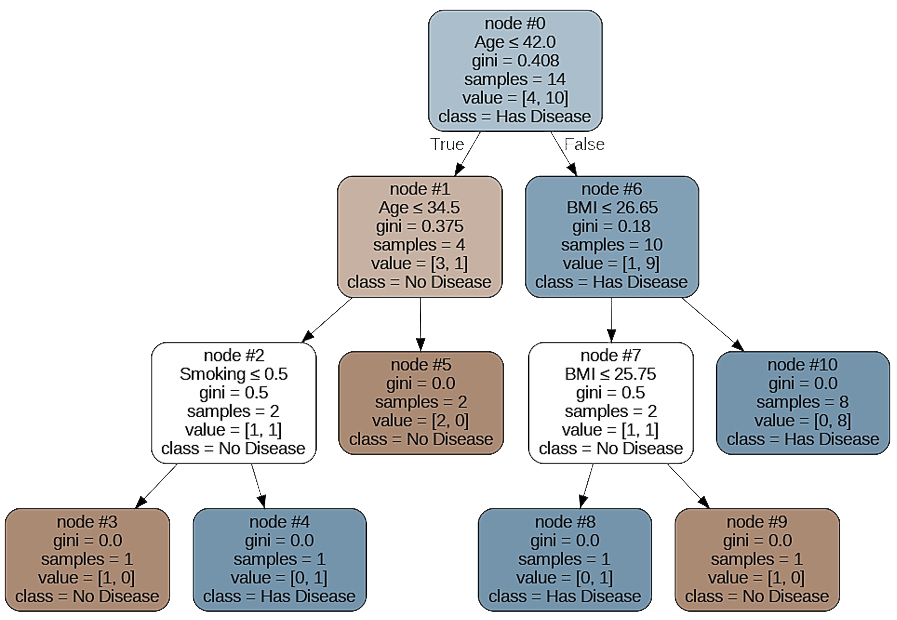
At the core of our mission is one key question: How can artificial intelligence transform the way we understand, prevent, and treat diseases? In our group, we aim to answer this by harnessing advanced AI techniques, such as Deep Learning, Bayesian Models, and Machine Learning, to extract meaningful insights from vast amounts of medical and epidemiological data. By applying AI to Electronic Health Records, epidemiological databases, and large-scale medical datasets, we strive to improve disease prediction, patient stratification, and treatment outcomes. Our research focuses on AI-driven solutions that enhance the accuracy of disease diagnosis, predict long-term health outcomes, and model the spread of infectious diseases. Ongoing projects include using AI for ECG-based prediction models of cardiovascular conditions, determining risk factors for neurodegenerative diseases such as Alzheimer’s, and developing interventions for Long COVID treatments. Through collaboration with clinical and public health experts, we integrate AI into real-world medical settings, aiming to bridge the gap between advanced analytics and tangible patient care improvements.
Machine Learning for Personalized Health
Personalized health is at the forefront of modern medicine, and our group is deeply involved in harnessing Machine Learning to tailor healthcare to individual needs. By analyzing genomic, clinical, and lifestyle data, our models can predict individual disease risks, treatment responses, and drug efficacy. We specialize in applying machine learning algorithms—such as Neural Networks, Clustering Methods, and Multivariate Regression—on patient datasets to offer precision health solutions.
Current projects involve developing predictive models for cancer classification, identifying genetic drivers of complex diseases, and machine learning-driven predictions for pharmacogenomic responses. Our work enhances precision medicine, offering personalized treatment pathways to optimize healthcare for patients based on their unique biological and clinical profiles.
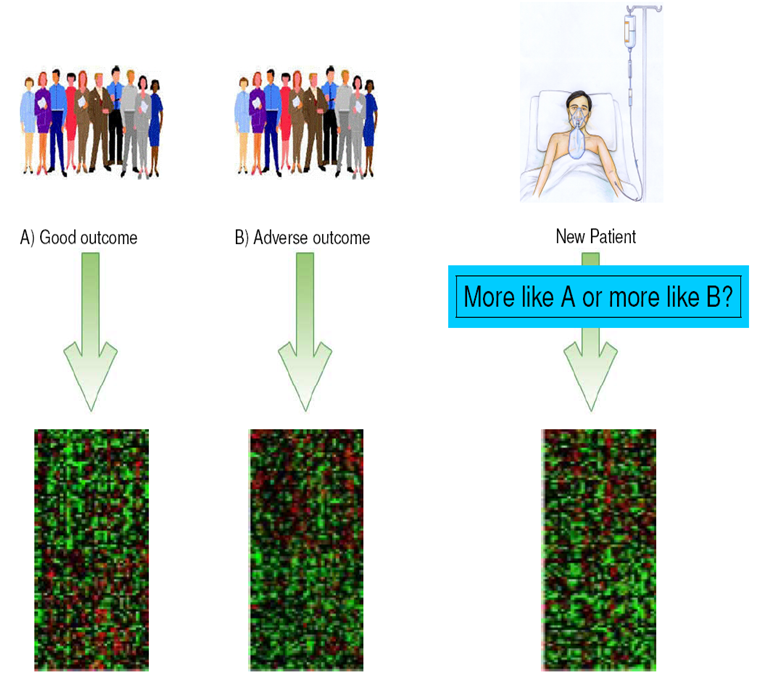
Network Causality in Biological Systems
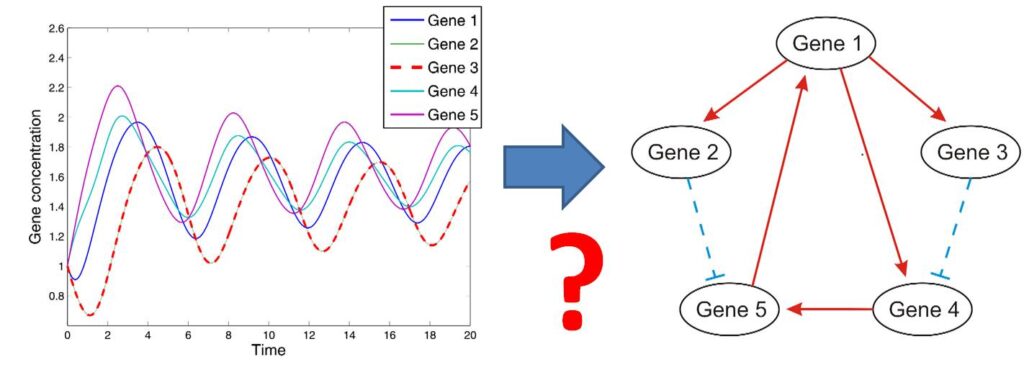
Understanding the underlying causal mechanisms in biological systems is a crucial goal in systems biology. Our group is at the forefront of developing methods for
Network Causality reconstruction, which involves the identification of causal relationships within complex biological networks. This approach is key for understanding how molecular interactions drive disease processes. We employ advanced computational techniques such as Bayesian inference, nonlinear dynamic systems, and machine learning to reconstruct these networks from high-dimensional data (e.g., time-series, interventions). Our focus extends to the study of gene regulatory networks, protein signaling pathways, and disease-specific networks (e.g., cancer and viral infections like SARS-CoV-2). These models not only reveal internal network dynamics but also provide insights into potential therapeutic targets, advancing precision medicine and drug discovery efforts.
Predictive Models for Precision Medicine
Precision medicine aims to predict, prevent, and treat diseases based on the patient’s unique molecular and genetic profile. In our group, we develop and apply advanced predictive models that go beyond traditional diagnostics. Using supervised and unsupervised learning methods, our models analyze large-scale genomic, transcriptomic, and proteomic data to identify disease biomarkers, predict disease progression, and optimize treatment strategies.
Key research focuses on predictive modeling for personalized cancer therapies, leveraging multi-omics data to identify individual genetic variations that influence treatment outcomes. We are also exploring the use of AI for pharmacogenomic analysis, allowing us to predict adverse drug reactions and select the most effective treatments based on a patient’s genetic background.