Transcriptomics and Genetic Epidemiology
Modern transcriptomics enables the comprehensive analysis of RNA molecules, offering insights into gene expression and regulation in various biological contexts. In our group, we combine transcriptomic data with genetic epidemiology to uncover how gene expression influences disease risk, progression, and treatment response.
We specialize in analyzing large-scale RNA-Seq datasets to investigate non-coding RNAs (such as miRNAs, lncRNAs, and circRNAs), their interactions, and their impact on gene regulation. Key projects include studying transcriptomic signatures in neurodegenerative diseases, intellectual disability, and cancer. Our work uses advanced computational pipelines, including Differential Expression Analysis, Gene Set Enrichment, and Pathway Analysis, to link genetic variation with disease traits at the transcriptomic level. This integration allows us to map the influence of genetic variants on gene expression across populations, paving the way for more precise biomarkers in genetic epidemiology.
Microbiome and Host-Pathogen Interaction
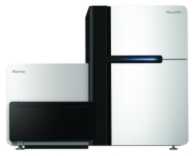
The human microbiome plays a crucial role in health and disease, influencing processes ranging from immune response to chronic disease development. Our group is advancing the understanding of how microbial communities interact with host biology and how pathogens contribute to disease mechanisms. Using state-of-the-art sequencing technologies such as 16S rRNA and shotgun metagenomics, we explore the composition of microbial communities in the gut, skin, and other tissues, as well as establish a reference microbiome for North-East Germany. Our research focuses on the interactions between microbiomes and pathogens, utilizing advanced computational methods and machine learning to uncover how microbial dynamics affect host physiology and their role in infectious diseases. We aim to identify diagnostic and prognostic biomarkers based on microbiome data and host-pathogen interactions, advancing the field of microbial genomics in disease prediction and therapeutic interventions.
Multi-Omics Data Integration and Biomarker Discovery
In the era of big data, combining multiple omics layers—genomics, transcriptomics, proteomics, metabolomics—offers unprecedented opportunities to understand complex biological systems. However, integrating and analyzing multi-omics data remains a challenging task. Our group is at the forefront of developing computational frameworks to integrate omics data for biomarker discovery and disease prediction.
We specialize in linking data from high-throughput platforms with clinical outcomes to identify molecular signatures of disease. By combining genomic variants, gene expression patterns, proteomics data, and more, we develop sophisticated models that allow for the discovery of actionable biomarkers. Our work in this area involves advanced machine learning algorithms to integrate diverse datasets and pinpoint molecular features that predict patient outcomes or treatment responses, particularly in cancer, neurodegenerative diseases, and cardiovascular conditions.
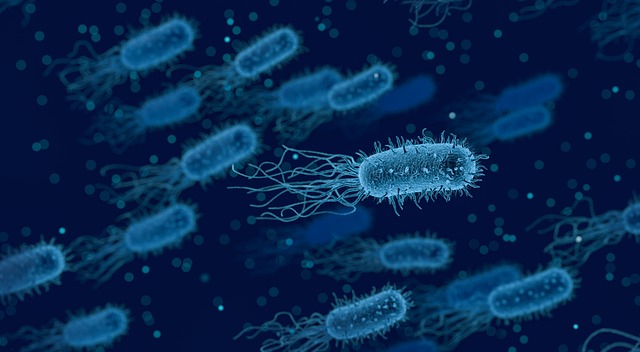
Multi-OMICs Data Integration
Integration of data stemming from different experimental platforms is becoming increasingly difficulty. Vast amounts of data are being generated, but interpreting and analyzing this data and integrating it with data from others studies and other platforms is becoming more and more problematic.
We develop and apply bioinformatics tools for multi-OMICs data integration, for example by integrating OMICs data with protein interaction and pathway information, thus mapping available experimental information to the underlying biological processes. Sophisticated computational algorithms are required for this task, to maximally extract information from experiments.
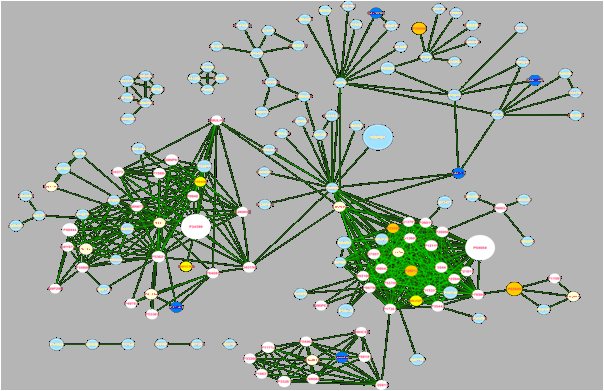
Molecular Consequences of Genetic Variation
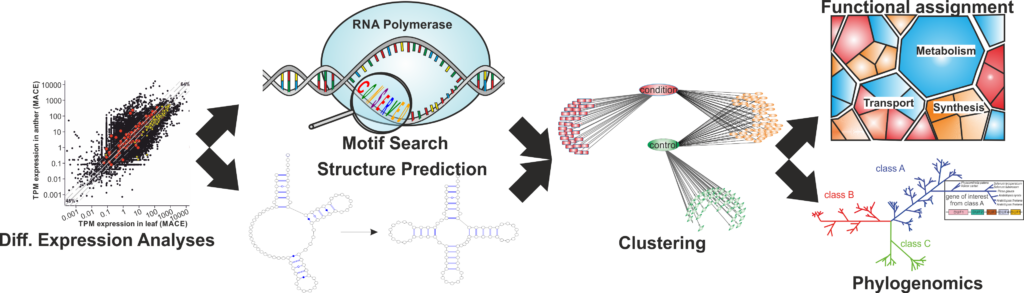
Understanding how genetic variation influences molecular mechanisms is critical for deciphering disease etiology and progression. Our group investigates the downstream effects of single nucleotide variants (SNVs), insertions/deletions (Indels), and structural variants (SVs) on gene expression, protein function, and cellular pathways.
We develop and apply bioinformatics methods to map genetic variations to functional outcomes, using transcriptomic, proteomic, and epigenomic data to explore how these variants drive changes in cellular processes. Projects in this area include exploring the genetic basis of vaccine-induced immune thrombotic thrombocytopenia (VITT), identifying genetic factors in cancer, and studying the molecular mechanisms behind age-associated diseases. Through computational approaches such as pathway analysis, network inference, and machine learning, we aim to elucidate how genetic variants contribute to disease phenotypes and identify potential therapeutic targets for precision medicine.